Times they are a-changin’ in predictive maintenance
Predictive maintenance, despite its measurable results and successes, has been suffering from lack of scalability. Models designed and trained for a given machine with a particular set of sensors often had to be retrained before being used with other similar machines. In addition, the quantity and variety of machines in a factory makes developing, training, and maintaining models for each machine a challenge. Therefore, it‘s natural that predictive maintenance became limited to the most valuable assets in a plant.
But that is quickly changing.
Technological advances have increased the availability of affordable vibration sensors, offered either with or without analytics solutions. New products come out often and in late 2020, the market heated up with the entrance of Amazon in the condition monitoring arena, with the launch of Amazon Monitron sensors. According to a research by Technavio, “the industrial vibration sensor market size is expected to grow by USD 2.83 billion during 2021-2025.”
And this is great news! With sensors being added to more machines, industries will be able to scale up predictive maintenance to entire plants and reap even more benefits. However, no change comes without challenges: the more machines are monitored, the stronger the pressure to collect data, analyze it, and quickly show assets that need closer inspection. To remain competitive, OEMs, plant maintenance companies, and sensor distributors will need to adapt by adding more analytics capabilities to their solutions.
That is why we are launching MultiViz Vibration. To enable companies and professionals that deliver machine diagnostics to add value to their offer through analytics features, powered by Machine Learning algorithms.
Traditionally, vibration analysis requires a vibration expert, detailed knowledge of the machines, and significant contribution from domain experts. This also contributes to the lack of scalability, especially in a situation in which all machines would have their own sensor.
Machine Learning becomes the solution to that, by allowing large-scale unsupervised analysis of the history of an asset or a population of assets. Additionally, our API makes it easy for asset health management, condition monitoring, and maintenance providers to build their own solution on top of it. Just connect data from vibration sensors or upload their own historic data and let our engine help with:
- Mode identification: narrowing asset data investigation with the identification of operating or failure modes based on periods of similar behavior over time.
- Black sheep detection: using population-wide anomaly detection to enable the prioritization of assets that require attention by comparing the behavior of similar machines and finding the ones that behave differently.
And more features are in the pipeline! We also provide a Python package for those who are not familiar with a REST API or would like to integrate it in their data analysis routine in Jupyter Notebook, for example.
Like the Bob Dylan song that inspired the title of this text says, times they are a-changin‘. Don’t wait for the walls to start rattling, click below to request your free trial of MultiViz Vibration and start making the most out of vibration data.


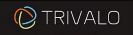
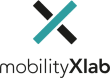
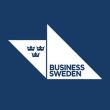
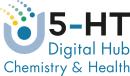
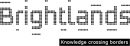
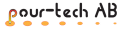
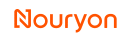
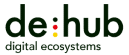
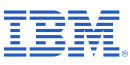
